PRM in a nutshell
Today, we opt to work on models instead of real systems in order to enhance their performance or to simplify many tasks such as prognosis, diagnosis, tests… etc. Several challenges are revealed on how to improve models’ expressiveness, i.e., when there is a lack in the system’s descriptive data, when there are problems related to the systems’ size or a presence of uncertainty.
In our case, we will specialize in modelling decisional systems specifically correlated with uncertainties; in order to cope with this task, the famous Bayesian Networks provide a powerful tool. However, despite the fact that BN has a simple and efficient implementation, it is still inadequate to deal with large scale real world. In simple words, BN lack both scalability and reusability; this leads the AI community to invest in order to enhance its performance. Several BN extensions appeared with the advent of object oriented programming such as Oriented Object Bayesian nets (OOBN), Multi Entity Bayesian Nets (MEBN), Probabilistic Relational Model (PRM)…etc.
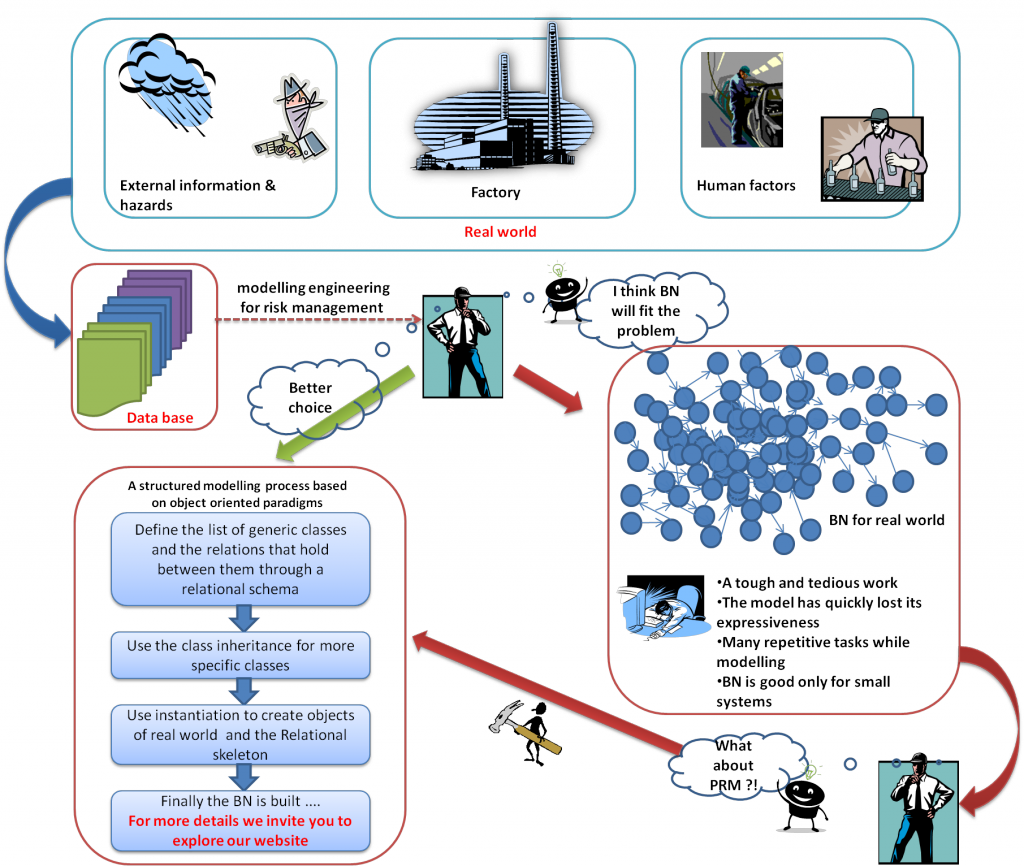